One of our financial services customers, a leading union-owned bank, needed to prioritize data governance and auditability as the pre-requisite before starting data modernization, advanced analytics and AI implementations.
The traceability of data flow at entity and attribute levels, calculations, and KPIs is a compliance need for them. Column level data lineage is not a common feature in many technologies currently. While different tools and technologies have their own pros and cons, a combination of Databricks and Fabric turned out to be a great choice for solving column level data lineage, centralized data governance and unified AI-powered data platform; Microsoft Purview provides overall data governance capabilities on top of it.
Databricks Unity Catalog Integration
This feature was in Private Preview when we evaluated the solution feasibility for this use case. Our solutions team was debating between using Databricks for granular data lineage vs. using third-party technology for this purpose and then integrating it with the Azure ecosystem. Databricks Unity Catalog integration with Fabric came out to be the natural best choice to solve this problem.
This option meant the solution could leverage all the great features of Fabric security, integrations, OneLake and data governance while bridging the gap of granular level data lineage using Databricks Unity Catalog.
Azure Databricks and Fabric: Well Integrated and Complimentary
In today’s complex and fast evolving data and AI space, a combination of compatible technology choice helps bring the best of the breed capabilities in the areas of big data engineering, BI & reporting, Data governance, machine learning, SaaS experience, AI capabilities, integrations, full-code vs low code and cost efficiencies. Azure Databricks and Fabric turned out to be that combination for the scenario like the above.
In this blog, we will drill down into a use case of end-to-end Data Flow with Unity Catalog, Transformations and Column-Level Lineage implementation using databricks, unity catalog and Microsoft Fabric.
Data Platform Context
Customer data landscape included business applications, databases on Azure SQL, ADLS Gen 2 lake and on-premises file systems as data sources.
Existing implementation lacked data lineage, especially the traceability of column level data flow for directly mapped as well as derived data fields and financial calculations / KPIs.
Our solution approach includes Fabric and Azure Databricks, along with Unity Catalog integration and Purview to accomplish end-to-end data platform, lineage and data governance. This blog focuses on Databricks Unity Catalog integration with Microsoft Fabric based data platform.
Implementation Walkthrough
In this section, we will walk through the step-by-step process of configuring and setting up Unity Catalog for lineage and integration with Fabric based data platform.
Screenshots show open-source datasets for technical illustration instead of actual customer datasets.
1. Configure Databricks Workspace
Create an Azure Databricks workspace with a premium pricing tier which is a pre-requisite for the Unity Catalog feature.
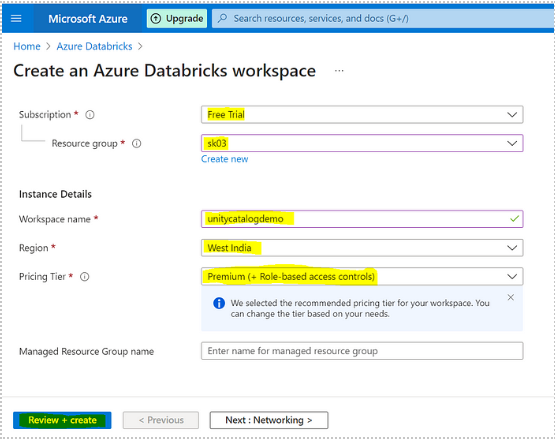
2. Setup of Unity Catalog, Creating Catalogs and Schema
To set up the Unity Catalog, make sure you have admin access and are using Databricks' premium or enterprise edition. Next, build a meta store. Navigate to the data tab, select meta store, and provide it with a name.
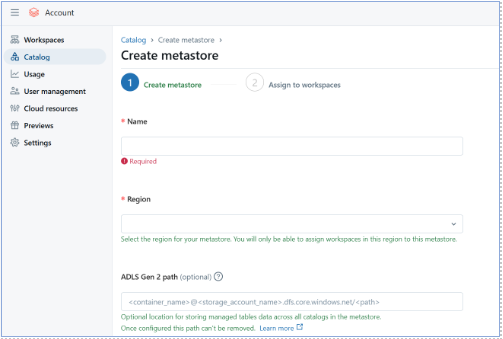
Select “Create”, and then “Catalog” option. Enter a name and description, then click “Create”.
Once the catalog has been generated, pick it from the Unity Catalog area. Click ‘Create’, then ‘Schema’. Enter a schema name and optional description, and then select a catalog. Click ‘Create’.
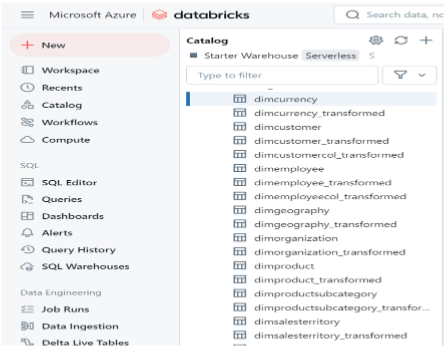
3. Mapping and Processing Data in Databricks
Ingestion of data from sources like existing data lake, Azure SQL and on-premises flat files using data bricks notebooks generates data lineage maps. This involves direct field mapping, data derivations, joins and creating KPIs in this example.
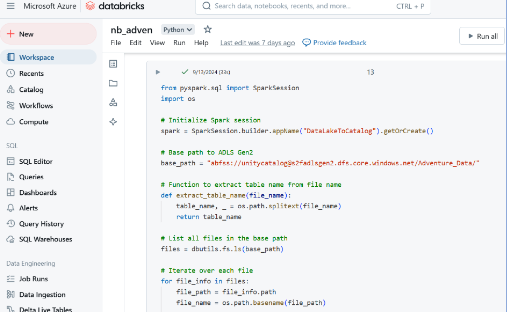
4. Tracking Granular Level Data Lineage
This process enables
- Use Unity Catalog’s lineage to track column-level data flows.
- Review and document the lineage for auditing and compliance.
Shown in the picture below:
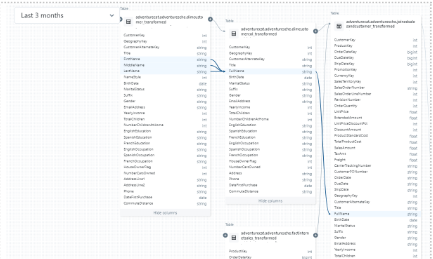
5. Writing Logs to Meta Store
Unity Catalog records all data access and metadata changes, including user actions such as reading and editing tables and schema. These logs provide full traceability of data usage and changes including user and timestamps.
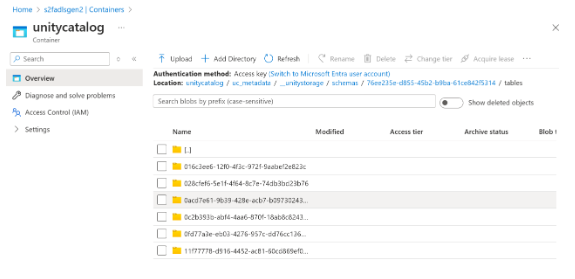
6. Integration with OneLake (Unity Catalog Shortcut)
Databricks shortcut will be a new artefact type in Fabric. When you create a shortcut for Databricks item, this creates 3 items in Fabric–
- Mirrored Azure Databricks item
- As SQL Analytics endpoint
- A default semantic model
To set this up, navigate to Data Warehouse experience in Fabric. We will select "Mirrored Azure Databricks Catalog" in the “Recommended items to create” section.
- Select "Mirrored Azure Databricks Catalog" in the "Recommended items to create" section or search for it.
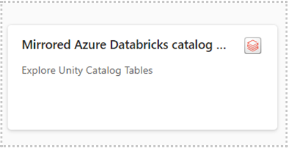
- Select an existing connection or create a new connection with the Databricks workspace URL as shown in the screenshot below.
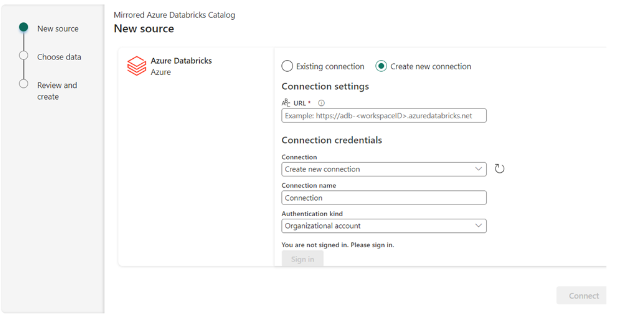
- Choose tables from a Databricks Catalog: By default, "Automatically sync future catalog changes for the selected schema" is enabled, reflecting metadata changes from your Azure Databricks workspace to Fabric.
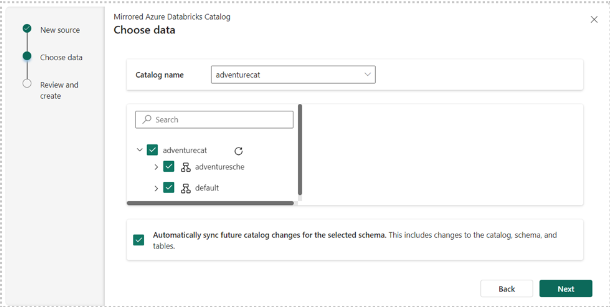
- Unity Catalog tables in Microsoft Fabric: You can preview data by selecting the SQL Analytics Endpoint, similar to other data shortcuts in OneLake.
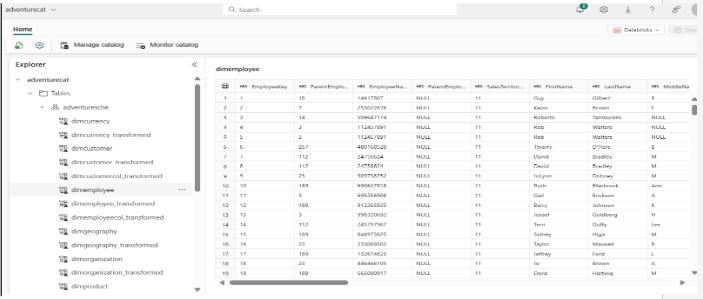
- Query Data: Access the SQL Analytics Endpoint Explorer page and query data using the SQL Editor. Finally, use the SQL Analytics Endpoint to examine the data and ensure that it is properly mirrored.
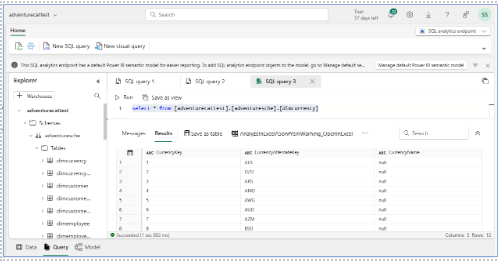
- Manage Semantic Model
After getting the data into the Lakehouse, you can review and manage semantic models and generate reports as with semantic models built on OneLake objects.
Below screenshot depicts the data flow from the ADB workspace to the Fabric semantic model
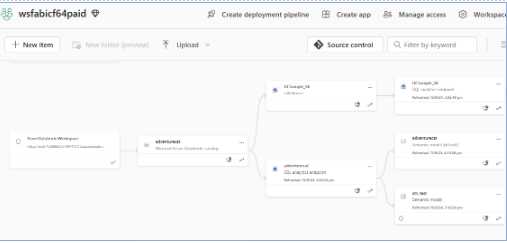
7. Turn off the ADB Cluster, the Lineage Data Continues to Work in the Fabric Environment
Once mirrored into the Fabric environment, ADB items are accessible in Fabric even if the ADB cluster is turned off. If you are processing data on Databricks side continuously, of course, you need to keep the clusters running and the refresh mechanism to Fabric scheduled to reflect the latest information.
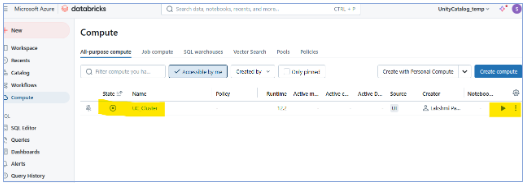
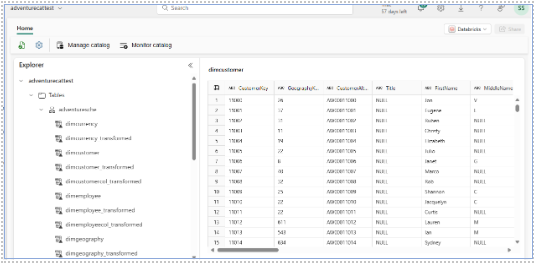
Security Considerations
- Currently, Unity Catalog integration into Fabric is not supported for the Databricks workspace that is behind the private endpoint. This is expected to be supported in the future.
- Storage accounts behind the firewall are also currently not supported.
- You do need to (and have an opportunity to) define data security in the Fabric environment for the shortcut objects; data security defined in Databricks is not automatically applied to the Fabric shortcut item.
Conclusion
Integrating Databricks Unity Catalog and Microsoft Fabric works well together to complement the capabilities of both technology platforms. This blog demonstrated the benefits of the ADB Unity Catalog and Fabric integration for a customer use case. Databricks can be plugged in to achieve fine-grained data lineage in the Fabric based data platform.
Related blog: European Fabric Community Conference 2024: Building an AI-powered data platform
Explore Sonata’s offerings for Microsoft Fabric here.
Authors:
Mahesh Hegde, AVP, D&A, Sonata Software
Contributor:
Syed Saida, Sr Data Scientist, Sonata Software